Fixing Music’s Samples Problem with AI: Leigh Smith on Selector
- marshallsoundz
- Sep 2, 2019
- 5 min read
A million sounds is a lot of sounds. Over-browsing is a huge problem when you’re trying to stay in your creative flow.
The new LANDR Samples helps you find the right samples fast with Selector’s AI sample recommendations. But how does it work? And can it solve the mess of endless choice?
We sat down with LANDR’s senior research engineer Leigh Smith to take a deep dive into LANDR Samples’s AI-powered recommendation tool—Selector.

Could you introduce yourself and tell us what you do at LANDR?
I’m Leigh Smith, I’ve been a senior research engineer at LANDR for the past four years, part of the team responsible for the AI research and development that drives LANDR’s products. I’ve been involved with the research and development of LANDR’s Selector sample recommendation system over the past 18 months.
I like to think of Selector’s recommendations as waves of possibilities.
What is Selector and what does it do?
Selector is LANDR’s AI driven recommendation of samples to users, a key component in aiding the expansion of our sample library available to our users. The principle behind Selector is to improve the musician’s experience of discovering and using samples in their creative projects. In addition to users searching for samples using traditional text queries, based upon labels of a sample, including such things as instrument name, genre, mood, tempo or key, Selector recommends samples based on acoustic similarity, a ‘sounds-like’ query, based on an existing sample the user has just listened to. In use, after a user has auditioned a sample, they can then find other samples which sound like the sample they just heard, which is intended to help them hone in or converge towards the sound they may have in their head, and are searching for, when browsing LANDR’s sample library. This sounds-like capability draws on the work that LANDR has done in our machine learning based automated mastering towards understanding the musically perceived qualities of digitized sound. We are using these psychoacoustic and musical features to index and recommend similar samples in Selector.
Why apply AI to a sample service like LANDR samples?
Sample library searching using text labels (or “metadata” as it’s known) is bounded by the need for people to have a shared vocabulary of labels that everyone understands and agrees with. Of course, that can either result in too many samples all having the same labels, or so many labels that it becomes hard for the user to know which label is appropriate to search for. This problem grows as more samples become available to audition and choose from. By adding an understanding of the actual musical audio signal that users are listening to, we are trying to help users more quickly find the sample that fits their creative needs, reducing the time they spend searching.
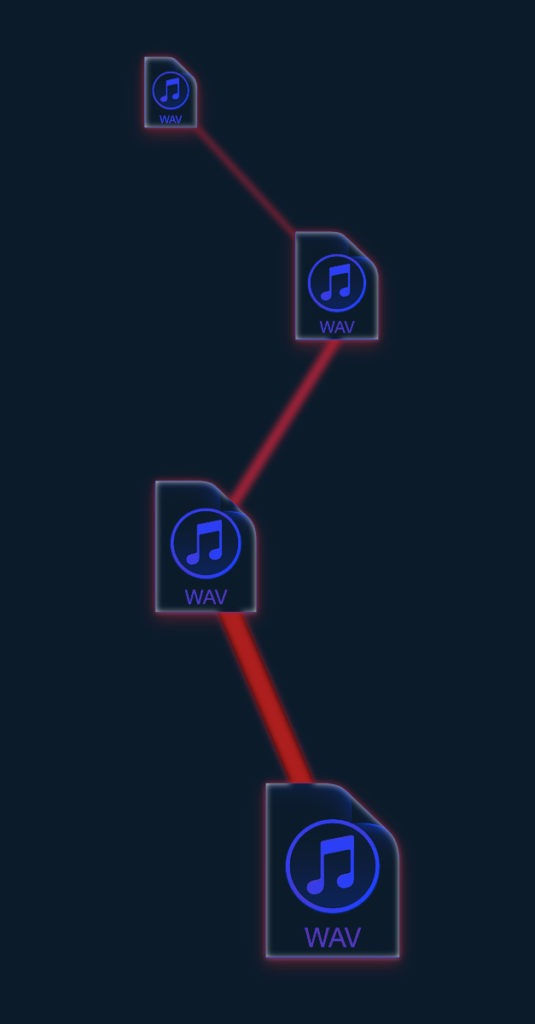
By adding an understanding of the actual musical audio signal that users are listening to, we are trying to help users more quickly find the sample that fits their creative needs, reducing the time they spend searching.
Is there a need for a boundary between the AI and the creativity of the musician?
In my opinion, that boundary arises naturally and changes as users engage with musical technology. It’s important to remember that AI is ‘artificial’, and I’m very confident that the creativity of musicians will continue to push the bounds as they can make use of a technology for their creative goals. Certainly our goal at LANDR is to support and enable our users incredible creativity, by leveraging AI to enable users to retain their sense of flow, and not get bogged down in trawling sample collections searching for that one sound which will be the keystone that realises their musical goal.
Do you think that AI sample recommendation could take away from the digger mentality of traditional sampling techniques?
We’ve tried to consider the goals of sample-based musicians, and add Selector’s recommendation in addition to text searching. I like to think of Selector’s recommendations as waves of possibilities, that you can surf from one to another, picking up on one recommendation from the list of recommended samples, and using that as another query, and seeing where that can go, jumping from a set of recommendations to the next. So that serendipity, from browsing or digging, is still there, but hopefully is a little more under the users control, based on their selections.
What problems does AI sample recommendation solve for musicians?
One goal is to reduce the time to find a sound that you have in your mind’s ear, allowing you to converge on your idea. The other, as I mentioned above, is to give musicians a way to explore our sample library in a way that is driven by sound itself, in preference to the typical modalities of vision or text. The other problem is that of addressing the scale of possibilities, as sample libraries increase, having a sense of what is actually available to musicians to use can be limited if exploration is limited to the names of packs, genres, moods or other descriptions. It also allows musicians to play directly with the idea of musical similarity, and to reflect on how similar or different they want a sound to be, compared to something they already have listened to.
Using AI as a means to aid searching is intended to reduce the friction between having the musical idea and realising it.
Is it possible to create entire tracks using Selector?
We’ve certainly had some of our in-house, and close collaborating, producers and musicians use Selector to start from a single inspiring sample, and to draw each subsequent sample to fit, building up entire tracks. They have found that choosing the degree of similarity, based on how far down the recommendation list they choose from, can be a way to express alternately unity or surprise in their choices of sounds, both of which are essential in building up a final track that creates an idea in the mind of the listener, and takes them on a journey of discovery.
Can AI make sampling more accessible to new and aspiring musicians?
We certainly hope so. The main way it benefits newer musicians, is it is relying on their listening and selection to help drive recommendations, reducing the need for them to be familiar with possibly obscure text labels to help them find what they are looking for. Using AI as a means to aid searching is intended to reduce the friction between having the musical idea and realising it, and when you’re starting out, this can make a big difference in moving you forward on a project.
Is there such a thing as too many samples and can AI help music producers struggling to sift through unlimited options?
The phenomenon of huge numbers of samples becoming available to every musician is unique to the current moment we live in. That offers a creative potential that didn’t exist for most musicians, even 10 years ago. But large numbers of samples alone doesn’t aid that potential – it’s how musicians can navigate these libraries and use them in creative ways which is critical. We are very keen to continue to learn from our users how they use Selector and other AI technologies in their creative process, and how we can improve those technologies to better help them in the future.
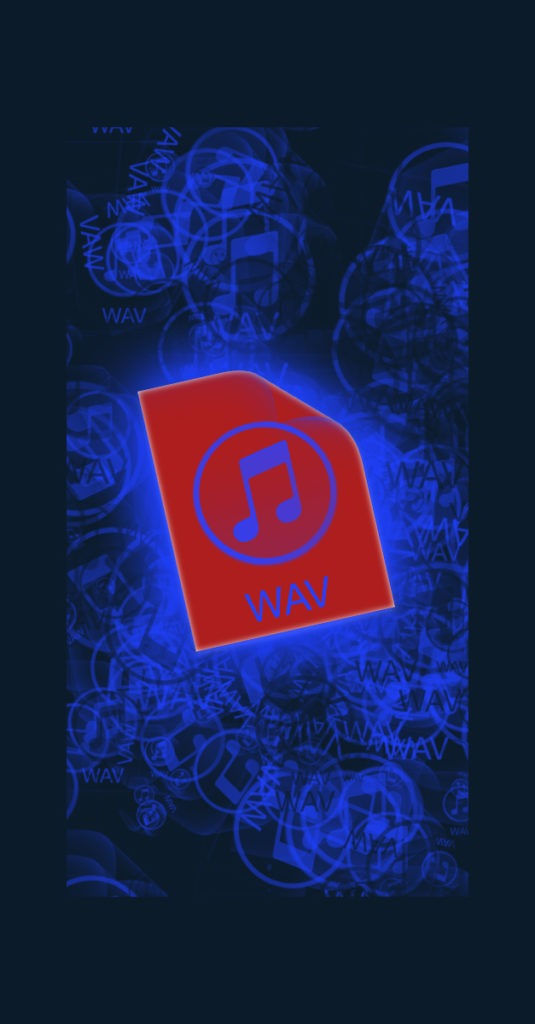
Browse the entire LANDR Samples Catalog now and discover the best sounds fast with Selector.
Comments